VGG-Face2 Mivia Ethnicity Recognition (VMER) Dataset
The VMER dataset is composed by images collected from the original VGGFace2, which is so far the largest face dataset in the world including more than 3.3 millions face images, with an average of about 362 samples per subject (minimum 87 images per subject). It also includes gender labels and consists of 62% males and 38% females.
In order to avoid the other race effect, we asked three people belonging to different ethnicities, namely one African American, one Caucasian Latin and one Asian Indian, to annotate each identity with an ethnicity label among the considered four.
To obtain the final annotations, we applied a majority voting rule, which allowed to determine the ethnicity label for 99% of the face images in the dataset; as for the remaining 1%, we employed a tie-break rule, by asking a fourth annotator the opinion about the ethnicity.
The final VMER dataset consists of 3,309,742 face images of 9129 identities. There is no subject overlap between the training and the test sets, namely the samples of the subjects used for training the networks are not included in the test set. In face analysis, this separation is very important for evaluating the generalization capabilities of the neural networks.
1) Download the VGG-Face2 dataset: http://www.robots.ox.ac.uk/~vgg/data/vgg_face2/
2) The file finalTrain.xml includes the ethnicity labels for the training set, while the file finalTest.xml contains the annotations for the test set
3) The labels are the following: 1: African American, 2: East Asian, 3: Caucasian Latin, 4: Asian Indian
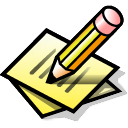
References
If you use these datasets please cite:
- Benchmarking deep network architectures for ethnicity recognition using a new large face dataset. Although in recent years we have witnessed an explosion of the scientific research in the recognition of facial soft biometrics such as gender, age and expression with deep neural networks, the recognition of ethnicity has not received the same attention from the scientific community. The growth of this field is hindered by two related factors: on the one hand, the absence of a dataset sufficiently large and representative does not allow an effective training of convolutional neural networks for the recognition of ethnicity; on the other hand, the collection of new ethnicity datasets is far from simple and must be carried out manually by humans trained to recognize the basic ethnicity groups using the somatic facial features. To fill this gap in the facial soft biometrics analysis, we propose the VGGFace2 Mivia Ethnicity Recognition (VMER) dataset, composed by more than 3,000,000 face images annotated with 4 ethnicity categories, namely African American, East Asian, Caucasian Latin and Asian Indian. The final annotations are obtained with a protocol which requires the opinion of three people belonging to different ethnicities, in order to avoid the bias introduced by the well-known other race effect. In addition, we carry out a comprehensive performance analysis of popular deep network architectures, namely VGG-16, VGG-Face, ResNet-50 and MobileNet v2. Finally, we perform a cross-dataset evaluation to demonstrate that the deep network architectures trained with VMER generalize on different test sets better than the same models trained on the largest ethnicity dataset available so far. @article{Greco_MVA2020,
author=”Greco, Antonio and Percannella, Gennaro and Vento, Mario and Vigilante, Vincenzo”,
title=”Benchmarking deep network architectures for ethnicity recognition using a new large face dataset”,
journal=”Machine Vision and Applications”,
year=”2020″,
publisher=”Springer”
}
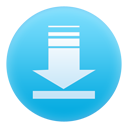
Download
In order to download the datasets click here.
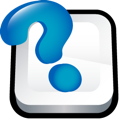
Help
If you have any problems, do not hesitate to contact us here.